CONCEPT DRIFT ISSUES IN RECOMMENDATION SYSTEM
An ideal learner should combine robustness to noise and sensitivity to concept drift Widmer and Kubat 1996. Concept drift and data drift.
Pdf Detection And Handling Of Different Types Of Concept Drift In News Recommendation Systems
The generic way to monitor concept drift is depicted in the following image.
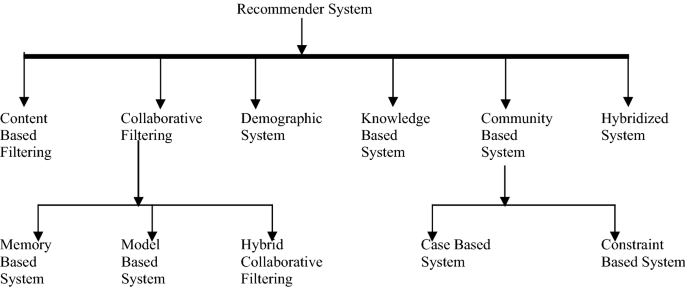
. Training new recommender models. This drift is a phenomenon that creeps into our models as time goes by and if we dont detect it on time it can have detrimental effects on our pipeline or services. Or new users and items may appear making the recommender system unable to accurately map the relationship between users and items due to the cold start problem.
Is referred to in the literature as concept drift 2 4 5. Concept drift detection and reaction in recommender. Concept drift usually refers to the change in the relationship between input and output data over time.
In turn the existence of concept drift requires RSs to adapt the user modelling process to the. Concept Drift-aware cloud service APIs Recommendation CD-APIR To adapt users preference drifts and provide effective recommendation results to composite cloud system developers we propose CD-APIR. Data modelli ng in IKCD.
First the training data set is collected and curated then the model is trained on that. Tackle concept drift early and often. CONCEPT DRIFT IN LEARNING PROBLEMS Relevant examples of learning problem in presence of Concept Drift includes.
Some algorithms may overreact to noise erroneously interpreting it as concept drift while others may be highly robust to noise adjusting to the changes too slowly. First the training data set is collected and curated then the model is trained on that. The model is continuously monitored against a golden data set which is curated by human experts.
1A real time recommender system. I do have dataset of users activity in an e-commerce website. In this article well take an in-depth look into model drift and explore two of its most significant causes.
Concept Drift IKCD algorithm a n adaptive unsupervised learning algorithm for recommendation systems in news data stream. They are usually affected by changes in the data distribution giving rise to a phenomenon referred to as concept drift. Considering the changes of user preferences especially the problem of concept drift the learning model for cloud service APIs recommendation faces a hidden technical debt issue ie the recommender system needs to continuously make new technical efforts to update the model to learn from new data Sculley et al 2015.
The generic way to monitor concept drift is depicted in the following image. Thus learning models must detect and adapt to such changes so as to exhibit a good. Recommendation systems and spam email filtering where learning task consists in predicting user preferences interests Financial market analysis where the learning task is to predict trends.
CD-APIR tracks users temporal preferences through users behavior-aware information analysis. Lets discuss a couple of scenarios that warrant contrasting approaches to handling drift. A difficult problem in handling concept drift is distinguishing between true concept drift and noise.
The model is continuously monitored against a golden data set which is curated by human experts. Up to 10 cash back Given that the anticipated recommendation should always meet user requirements while also gaining a better understanding of what interests a broad range of users we identify several emerging research aspects that will benefit from future research on recommender systems. Lets say we have a sequence of.
Two key problems result from concept drift. Concept drift causes the output recommendations to skew from the current user interest over time and become irrelevant 6. Moreover well shed some light on why they happen their implications how.
First the algorithm starts making predictions that are less accurate often much less accurate. Data stream mining extracts information from large quantities of data flowing fast and continuously data streams. This problemis referred to in the literature as concept drift 2 4 5.
An example of Concept drift is more likely in an email recommendation system as you discover enhancements from the production data set that were not considered with the test dataset. Concept drift is healthy but it should not disqualify your efforts or heavily constrain your timeline to deployment. Concept drift monitoring system.
Although the traditional techniques of RS have attained significant success in providing recommendations they are insufficient in providing accurate recommendations due. One of the major issues facing RSs is that the users interest andor the item features themselvesmay change over time especially for data streams that online users interact with 4. Singular Value Decomposition SVD and Jensen.
So it might recommend a shipping route thats slow costly or even dangerous. Nonetheless users preferences may change over time the so-called concept drifts. You would want the model to evolve as quickly as possible adequately.
Consequently concept drift and cold start are challenges that downgrade the recommender systems predictive. Concept drift monitoring system.
Pdf Factors Affecting The Performance Of Recommender Systems In A Smart Tv Environment
Evolution Of Recommender System Over The Time Springerlink
Continuously Improving Recommender Systems For Competitive Advantage Using Nvidia Merlin And Mlops Nvidia Technical Blog
Advances And Challenges In Conversational Recommender Systems A Survey Sciencedirect
Applied Sciences Free Full Text Recommender System Based On Temporal Models A Systematic Review Html
Informatics Free Full Text Exploiting Rating Abstention Intervals For Addressing Concept Drift In Social Network Recommender Systems Html
Applied Sciences Free Full Text Recommender System Based On Temporal Models A Systematic Review Html
Applied Sciences Free Full Text Recommender System Based On Temporal Models A Systematic Review Html
Recommendation Letter For Student Scholarship In 2021 College Recommendation Letter Reference Letter Letter Of Recommendation
Belum ada Komentar untuk "CONCEPT DRIFT ISSUES IN RECOMMENDATION SYSTEM"
Posting Komentar